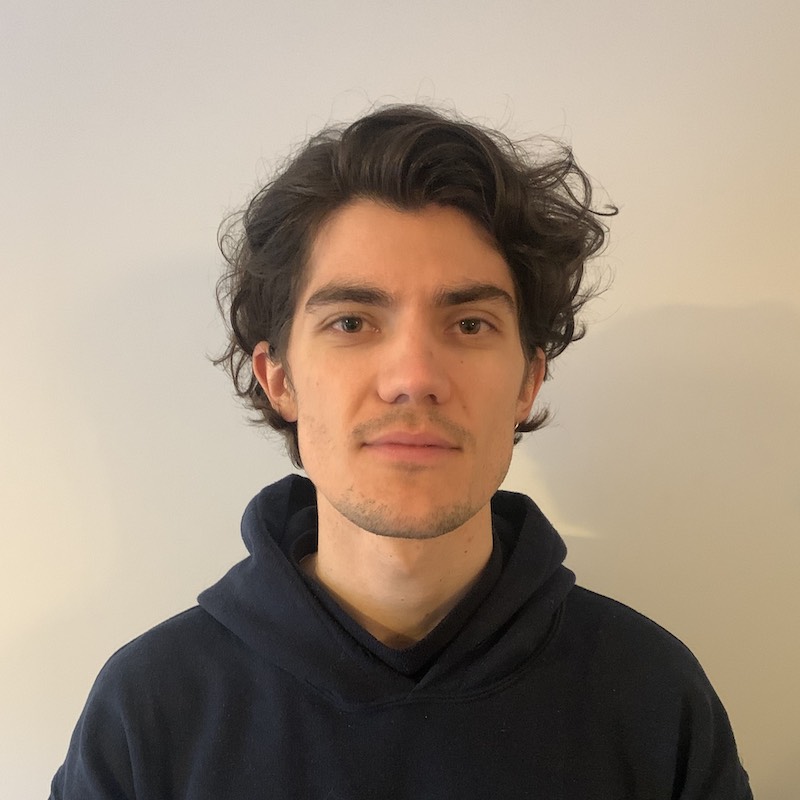
Dr. Lawrence Bull
11:30 21 August 2024
University of Glasgow | Mitchell Fellow in Statistics & Data | Website
Optimal Signal Reduction by Maximum Mean Discrepancy
The Maximum Mean Discrepancy (MMD) is used widely in machine learning to approach the two-sample problem - favoured for its interpretability and robustness to outliers. However, the MMD is not without caveats. As is typical for kernel methods, it suffers from the curse of dimensionality, which becomes problematic when monitoring high-dimensional data, often associated with anomaly detection for security applications. In this work, we formulate a procedure for the compression and decorrelation of high-dimensional signals, to enable robust change point detection in a decoupled space via the MMD discrepancy. In other words, we solve an optimisation on a manifold to learn a projection matrix that maximises potential information gain between compressed signals. Motivated by security applications (fraud detection) we demonstrate how the approach enables interpretable change-point detection, presenting the algorithm and its implementation in the auto-differentiation software jax.